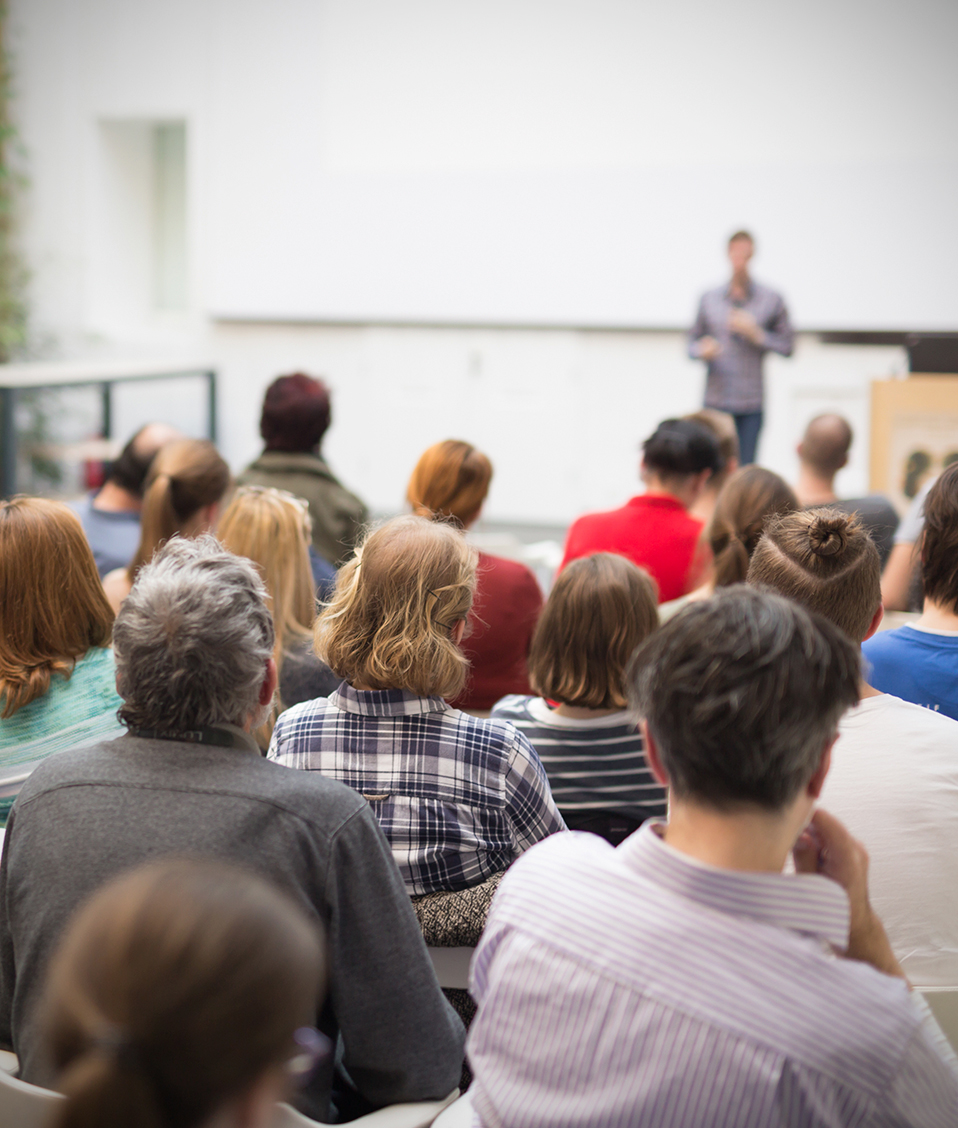
Conferencia 1: 12 horas día 4 de octubre
Título: Multivariate experimental errors in Principal Component Analysis: from correlations to loadings
Conferenciante: Edoardo Saccenti, Associate Professor. Laboratory of Systems and Synthetic Biology, Wageningen University & Research, the Netherlands
Abstract:
Multivariate measurement errors are ubiquitous in all experimental sciences. Depending on the experimental platform used to acquire data, diverse types of errors are introduced, amounting to an admixture of additive and multiplicative error components that can be uncorrelated or correlated.
In this talk I will review how experimental error affect correlations, the building blocks of multivariate statistical methods, and how this connect to the recovery of the subspace with Principal Component Analysis (PCA) as obtained by using numerical simulations. I will how show different error characteristics (variance, correlation, correlation structure), loading structures and data distributions influence the accuracy to estimate an error-free (true) subspace from sampled data with PCA.
Biography:
Dr Saccenti interests are the development of (interpretable) data analysis tools for the integration and analysis of large data sets and their application to solve complex biological and biomedical problems. He has interests in principal components analysis and related component methods with a focus on the problem of dimensional assessment and its relationships with inferential statistics, power analysis and sample size determination in the context of principal components analysis, partial-least square discriminant analysis and network inference; he does so by combining classical multivariate approaches with machine learning and recent tools from artificial intelligence and deep-learning methodologies.
He develops statistical and chemometrics methods in the context of system medicine, systems biology, metabolomics and bacterial genomics. He is an expert in the analysis of biological data stemming from high-throughput measurement techniques like NMR and Mass-spec. His applied research mostly focuses on health application in both humans and animal and bacterial infection.
He is author of more than 104 peer-reviewed scientific articles and book-chapters, totaling more than 6000 citations. His H-index is 37. https://scholar.google.nl/citations?user=W4G8JkkAAAAJ&hl=en
Conferencia 2: 12:45 horas día 4 de octubre
Título: Multidimensional Latent Path Models
Conferenciante: Age K. Smilde. Emeritus-Professor of Biosystem Data Analysis, Swammerdam Institute for Life Sciences, University of Amsterdam and professor of Computational Systems Biology at the Department of Plant and Environmental Sciences at the University of Copenhagen.
Abstract:
In many cases in the life sciences we are confronted with measurements performed on a biological system that can be arranged in multiple data sets pertaining to different entities being measured, such as metabolites, proteins, gene-expressions, microbiome compositions. Traditionally such multiset data has been analyzed by multiblock or multiset methods from chemometrics and psychometrics. Such an analysis can be supervised, e.g., for predicting an outcome, or unsupervised, e.g., exploratory analysis. Both types of analyses ignore the topology of the individual data blocks, i.e., the way in which these individual data blocks can be or must be arranged.
In the social sciences methods have emerged combining factor analysis with structural equation models resulting in so-called latent path models, such as PLS-Path Modeling, that deal with such topology. These latent path models usually assume one latent variable per data block which is not sufficient for high-dimensional life science data. In this talk, I will discuss how this can be generalized to more than one latent variable per block. This is not trivial and I will discuss methods, algorithms and interpretation of such models. I will touch upon the topic of causality and will give an example from the field of plant sciences.
Biography:
During the last three decades, Prof. Smilde has been a principal actor in the development of multivariate analysis in chemical and biological applications in system biology, metabolomics and the chemometrics area, in which according to google scholar is the fifth top researcher worldwide. His vast research work is outstanding, presenting an H index equal to 78. Prof. Smilde is co-author of more than 300 publications mainly related to the use of multivariate analysis in life sciences applications. His contributions to the use of three way analysis, data fusion and analysis of variance are of high impact. In three-way analysis, his book "Multi-way Analysis: applications in the chemical sciences" is a widely recognized monograph. He is co-author of state-of-the-art cross-validation papers on widely used multivariate techniques, like Principal Component Analysis and Partial Least Squares-Discriminant Analysis. He is author of the Anova-Simultaneous Component Analysis (ASCA) technique, an ANOVA-like technique that is experiencing an exponential growth in use.